DeepBrainResonator: cutting-edge EEG Deep Learning algorithms.
We are made up of trillions of cells not least of which is one hundred billions neurons contained in the human brain. These neurons serve for proper operation of our nervous system and consequently information communicating from our brain to the rest of our body organs. In case our neurons, or nerve cells, get affected or invaded by an ill factor, this may have a morbid action upon our whole organism.
With regard to the future and health preserving we created a breakthrough product for medical diagnostics automatization – DeepBrainResonator has turned clinical electroencephalogram (EEG) diagnostics around and brought hope for those who suffer from brain and seizure disorders, such as epilepsy, encephalopathy, encephalitis, brain tumor and sleep disorder.
The choice of automating EEG analysis is conditioned by complexity of analyzing the electrophysiological readings data. It stands to mention that short EEG records normally take 3 to 24 hours, and long records even up to 5 days, whereas patients are required to stay in hospital at all times.
Together with our partners we analyzed huge amounts of clinical data in order to gain knowledge from human brain rhythms for the clinic to improve diagnostic efficacy and accuracy. Though it had been impossible for a long time since the lack of large databases and suitable methods for exploiting them, we succeeded to create the first EEG analysis software based on the latest Deep Learning methods (Artificial Intelligence) due to access to clinical data provided by CSS partner – world renowned clinical neurophysiologist Professor Michel van Putten.
There is no gainsaying that The DeepBrainResonator project is a unique brainchild of fruitful collaboration and partnership between data scientists, entrepreneurs, software engineers and medical doctors.
Who are the creators of the breakthrough in EEG diagnostics?
The DBR project is carried out by a well-fitted team of four partners highly proficient in their expertise fields:
- Foremost, the project leader – MEDrecord – provides a semantic interoperability platform that serves as the underlying platform. It is designed for better care coordination and clinical diagnostics improvement in health facilities and is completely certified for ISO27001, NEN7510 en MedMij, and it helps startups and institutes for the fast development of validated apps.
- Clinical Science Systems (CSS) along with the partner Professor van Putten made substantial contributions into extensive clinical data research based on the Deep Learning method. CSS together with the professor have extensive experience in clinical EEG interpretation and developing EEG tools.
- Additionally, the Austrian Institute of Technology (AIT) developed EEG and ECG analysis software licensed in commercially available medical devices. The Deep Spike Algorithm developed by AIT outperforms all previous approaches for detection of epileptic discharges. AIT developed an algorithm for automatic detection of EDs, based on a novel deep learning method that requires a low amount of labeled EEG data for training. Detected EDs are automatically grouped into clusters, consisting of the same type of EDs, for rapid visual inspection.
Overcoming manual EEG challenges
As we know, prevention is better than cure, whereas healthy sleep structure is the most reliable ally in preventing numerous major and minor neurological diseases. Epilepsy, being the most common therapeutic indication for EEG, is no exception.
Long-term EEG monitoring is the most accurate diagnostics method, it enables not only high precision diagnosis of a patient’s brain or seizure disorder, but also control of treatment response when studying human sleep structure. In light of this, we concentrated much efforts on automatic interpretation of sleep stages based on EEG recordings. The results showed that automatic evaluation methods were able to perform a robust analysis of the brain activity recording.
For the reason that EEG is an indispensible stage in epilepsy diagnosis, we also laid down an aim to develop an automatic algorithm to detect interictal discharges, and it has been accomplished – DeepBrainResonator overcomes limitations in disease detection performance, it saves clinicians’ time and efforts while interpreting the results.
Please check the 2 minutes video:
What makes the project a milestone in EEG Deep Learning
Two-years long arduous toil of studying the diversity data and enormous number of samples per use case made training of DeepBrainResonator’s deep neural networks possible. Having conducted an in-depth study, the project developed three main deep learning-based algorithms applicable and fundamentally new to medical diagnostics.
Importantly, the ‘detection of interictal discharges’ and ‘detection of epileptic seizures’ algorithms allow for shortening of computation time previously spent on 24 hours EEG decoding, which is one of the primary parameters for a server based application like DeepBrainResonator is.
The third algorithm – ‘sleep staging’ – provides pinpoint accuracy and reliability, particularly compared to those of manual performance, which is a highest importance matter in EEG interpretation. In this way, DeepBrainResonator automates the visual assessment of EEG data by a clinical expert and facilitates interpretation quality.
Apart from that, DeepBrainResonator is an optional stand-alone software package that can be integrated with most of existing workflows and packages (Epic, iSoft, Philips, Siemens, etc.), and it does not need any kind of additional supporting software which is unconditionally prioritized by the system when choosing an application.
These main parameters ensured the DeepBrainResonator project success in the Electronic Health Records area, standardization and security on par with making the software an irreplaceable assistant in diagnostics, treatment and prevention of countless neurological disorders.
Lessons learned
Main thing what we learned was that we have been working with the best of best, so on the technical side it actually went rather smoothly.
What we have also learned from this innovation is any invention being highly dependent on human acceptance. No wonder the DBR success is largely predetermined by neuroscientists approval. And since the invention we have established inside this project is considered to be more like a competitor to the neuroscientists, the acceptance tends to be rather low. This aspect is rather intractable and not easily solved, it is otherwise known as ‘NIH’, or ‘not invented here’ syndrome.
Funding
The budget of almost two million Euro was funded by 45% by the Eurostars scheme with Grant agreement E!12398. The remaining one million Euros (55%) was invested by the individual partners themselves. See the RVO project page.
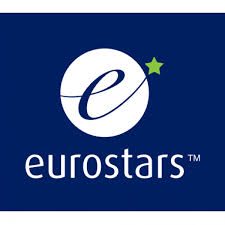